Data Engineering is a vital first step on the road to any successful analytics project. Our Data Engineering Practice Lead, Joyjeet Nag, explains why it’s so essential.
It’s every company’s goal to serve its customers better. And today, data-driven insights are an invitation to do just that.
As compute power has increased over the years, more and more organisations are turning to analytics to help them understand customer needs and market trends – and using that knowledge to provide better, more personalised products and services.
Analytics can provide visibility into any number of things, from where best to allocate marketing budgets to how to price different products at different times. But, no matter what it is you’re trying to uncover, analytics work can only be as good as the foundation it’s built on.
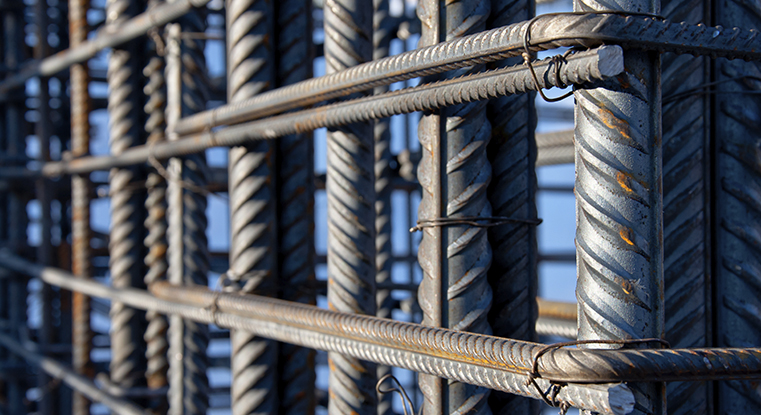
That’s where data engineering comes in. Data engineering is a process that provides the requisite tooling and technologies to transform vast amounts of historical data into a useful format. And by sourcing, transforming and analysing data from each of your systems, it ensures you can extract the insights you need, as and when you need them.
For those eager to make the most of more data-driven working practices, and generate new insights as quickly as possible, this part of the process is often overlooked. In fact, some organisations are entirely unaware that they need to take these steps to begin with. But without data engineering, there’s a good chance your analytics project will struggle to deliver value – and that means huge opportunities can be missed.
The big challenge of organising your data
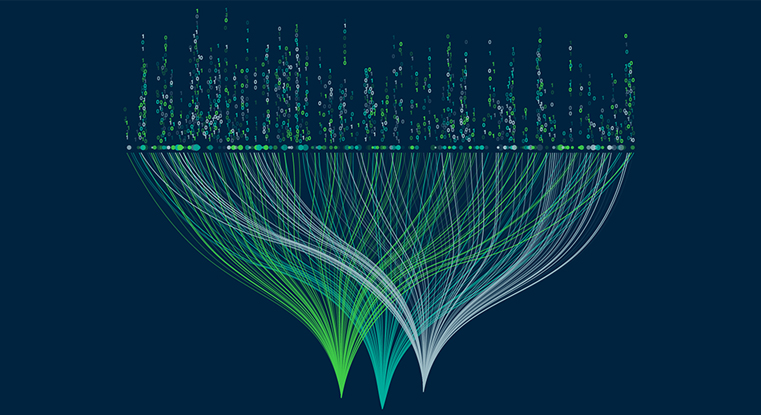
Data engineering isn’t a straightforward process, and it can present a number of challenges for organisations without the required in-house expertise. Large organisations especially tend to have vast amounts of historical data, which means those tantalising analytical insights aren’t always so easy to find. It’s the proverbial needle in a haystack, and finding your needle requires navigating huge data silos to ensure data is available, complete and ready to be mined for insights.
The ultimate goal of a data engineering process is defining the right approach to get that all-important ‘single view of the customer’, and there’s a lot of background work that goes into that. You need to know what systems you’re going to use to source data from, and what that complete dataset should look like – because if your data is missing vital components then the insights you’re reaching for will always be just short of your grasp.
You also need sufficient controls in place to ensure the quality of your data, and to make sure it provides value on a consistent basis. That requires dedicated data owners with clearly defined roles to ensure your data is up to standard.
For those who invest the time upfront, all of this effort will be repaid later down the line. But we still regularly see organisations approach this subject as an afterthought. In many cases, they’re all too keen to get to the perhaps more exciting analytics work, to stop and ensure their houses are in order before they begin.
This results in inaccurate insights, multiple, conflicting versions of the truth, and a failure to achieve the clarity of decision-making that was the initial impetus behind the project.
What do you need?
In our experience, almost every company we work with has different data engineering needs. There’s no magic bullet solution, which is why the approach we take is customised to every engagement.
Fundamentally though, our goal with data engineering projects is to make sure that our clients have a trusted source of data to work from. After all, good, timely, reliable data is the beating heart of any analytics project.
This can involve us identifying the right sources of data and defining quality standards to establish what a complete customer dataset should look like.
Or it can mean helping to select the right tools and solutions to meet strategic goals. This is often an interesting process, as most data engineering solutions are built on the technology our clients already have in place – whereas we are able to look at a business problem with fresh eyes and establish the best solution from a more impartial standpoint.
Ultimately, we provide whatever it takes to get your house in order and ensure you’re analytics-ready. This all begins by understanding your data ecosystem, needs, and current level of maturity before we work on identifying and deploying a custom solution that will ensure successful data and analytics projects for years to come.
As data engineering is just one step in the analytics journey, we also place a lot of emphasis on a continuous and collaborative relationship between data engineers and data scientists.
This is vital to ensuring scientists are sourcing data from the right places and using the correct attributes for the correct tasks. To this end, our data engineers will meet regularly with data stewards and data owners to make sure these teams work hand in hand.
If this process is cemented early enough, you can have complete trust in your data and the outputs of your projects.
The tangible value of data engineering
At The Smart Cube, we combine human intelligence with the latest technologies to help organisations in all industries and sectors deliver end-to-end analytics services – from data engineering to analysis and visualisation.
Recently a major international CPG client sought our support to set up its Data Engineering base in a Western European country. This required us to organise a book of work, dictate development processes, and establish tooling requirements, standards, and processes to ensure that all the data needed was ready and available.
We were then tasked with driving an initiative to adapt a US-based market mix model to work for European markets – leading the deployment and rollout of the model from the data engineering stage (with challenges arising from the European data having different levels of segmentation) – all the way to the data science work that delivers insights.
Through identifying inefficiencies in processes and introducing automation, we’ve been able to significantly reduce the amount of time the client spends validating data – resulting in efficiency gains of around 60% and up to 40% faster time-to-market.
If you’d like to see how we can help you with your data engineering – or any other stage of your analytics projects – don’t hesitate to get in touch.