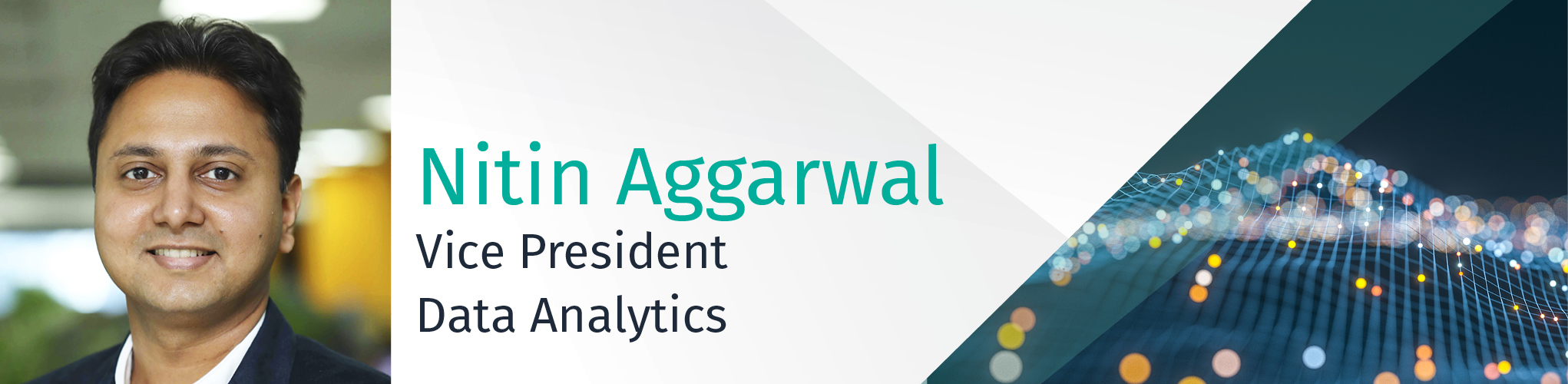
“I think there’s too much noise and confusion in the market around AI. It can make it difficult for organisations to understand what ‘artificial intelligence’ actually looks like for them.”
With over fifteen years of analytics experience under his belt, vice president and industry lead Nitin Aggarwal has seen the definitions, uses, buzz words and technologies that underpin data science change dramatically – and he believes that’s introduced some confusion to the industry.
Throughout his career, which started with data and computer algorithms at Computer Sciences Corporation, Nitin Aggarwal has worked with analytics in its many forms for over fifteen years.
“I was lucky to be exposed to lots of different types of analytics from the very beginning,” says Nitin, who’s now The Smart Cube’s vice president and industry lead for analytics in the retail, CPG and consumer markets. “This was especially true in my strategy development role at US department store chain Sears Holdings, where I started as an end-user, before moving into roles where I commissioned analytics projects, and eventually I ended up building the solutions myself.”
During his career, Nitin has witnessed rapid evolution across data, analytics and underpinning technologies: “In this industry, you always need to pick up new skills. There is a far greater volume of data and diversity of data types than ever before, plus a dynamic tech landscape to match. All of this is continually evolving, so we have to be able to identify the right sources and types of data, and learn to use the advanced analysis techniques and specialised tools, to get clients the insights they need.”
Defining innovation
As analytics tech changes so quickly – and dramatically – Nitin has noticed a potentially confusing trend around one of the most promising technologies of our age: artificial intelligence (AI).
“I think there’s too much noise and confusion in the market around AI,” he says. “It’s driven primarily by the marketing buzz, which can make it difficult for organisations to understand what ‘artificial intelligence’ actually looks like for them.”
With so many different technologies and services carrying the ‘AI’ label these days, it’s not always clear exactly what that might mean. In the coming 12-18 months, Nitin expects greater demarcation between AI and analytics to emerge, and hopes that providers will define their offerings more clearly: “The innovation we’re seeing is all important and necessary, but we need more clarity around exactly what AI is. That also applies to data science, another term being used very loosely across the industry, because organisations have different ideas about that, too.”
This feeds into a broader point Nitin has identified from his experience as the data analytics practice lead: the idea that ‘state of the art’ isn’t the same for everyone. It’s easy to define new and innovative technologies as state of the art, but for some companies, it will be beyond their requirements and capabilities at their current stage of maturity.
“For companies at an early stage in their digital journey, a carefully managed spreadsheet with automated formulas might be exactly the level of sophistication they need,” explains Nitin. “But for an organisation that’s at a higher position on the maturity curve, a spreadsheet would feel medieval. These firms will typically have enterprise BI (Business Intelligence) programs, as well as some use cases based on predictive models and machine learning algorithms.”
True AI-driven organisations, those with a high level of digital maturity and a clear AI strategy, are the ones that are experimenting with and implementing deep learning and advanced machine learning algorithms at scale – ‘the real AI work’, as Nitin calls it. Smaller or less mature organisations often focus on these exciting technologies, and worry that they’re missing out, but it’s important for them to recognise there’s still major value to be gained from more basic data analytics practices.
Charting maturity at an industry level
It’s not just individual companies that vary in their data maturity levels, Nitin notices: there are differences in maturity both at an industry level and in sub-segments of industries.
“Defining different levels of maturity is difficult,” he says. “Broadly, industries such as retail, CPG and healthcare are more advanced than others, like education – but there are differences at a more granular level that we need to understand.”
Take supermarkets as an example. With extremely high competition, razor thin margins and no significant way to differentiate their offerings, most large supermarket chains were early adopters of data science and analytics. Now at a high level of maturity, these companies are improving customer experience using computer vision and video analytics, managing shelf availability through neural networks, and predicting buying behaviour at a very granular level.
Compare that to a high street apparel store, which competes much more strongly on the trends it follows and the style of clothes it sells. With an offering that’s comparatively easy to differentiate from other stores, analytics maturity tends to be lower than that of a supermarket, for example.
Some companies have even found themselves relatively insulated from the industry changes that have driven other organisations to embrace data science and analytics, as Nitin explains: “If you look at food processors that supply to food and beverage companies, their business hasn’t changed in decades. Often, they’ve gotten comfortable, and without challengers they haven’t had the need to differentiate using data analytics.”
There’s always something new to learn
For Nitin, the variety of roles and industries he’s been involved in since the start of his career has been vital to his development.
“It’s been a great learning experience – understanding all the different types of analytics and what they can do for clients,” he says, “You need to have a wide range of functional and technical knowledge to deliver on so many unique projects, but you’ve got to be ready to learn, too. In fact, I believe success largely depends on one’s ability to un-learn and re-learn.”
During his time at The Smart Cube, Nitin has led a 150-strong delivery team, across markets, with analysts based around the world. This diverse team worked on many different projects, each of which needed a unique combination of business knowledge, technical skills, tools and resources – which he was responsible for orchestrating.
“The opportunity to work on such a diverse set of business problems is both challenging, and so intellectually stimulating,” he adds.
In fact, Nitin’s favourite part of the job might not be what you’d expect: “I find the proposal stage very interesting – it’s the point where you’re confronted with all sorts of new problems, and need to think up unique ways analytics can help.”
Want to meet more of The Smart Cube’s data scientists? Keep an eye out on our blog over the coming weeks and months!