Next in our blog series exploring interesting analytics use cases, we examine how machine learning algorithms dictate the music we listen to every day.
In 2019, the music streaming market was valued at $12,831.2 million – a figure that’s expected to nearly double by 2027. Music streaming has become the most popular medium for music consumption, significantly outperforming physical and digital sales since 2015.
But unlike previous jumps from vinyl to cassette or CD to digital purchases, music streaming has fundamentally changed consumers’ listening behaviours and their relationships with the music they listen to. And it’s all thanks to big data analytics.
Major platforms like Spotify, Apple Music, YouTube Music, and Tidal have near-perfected the algorithms they use to create highly accurate, personalised music recommendations for their users. These algorithms blur the line between the music you discover naturally, and the music that’s been strategically picked for you.
Here’s how music streaming platforms get their recommendations so right – and how it’s impacting the music industry.
More data, more accuracy
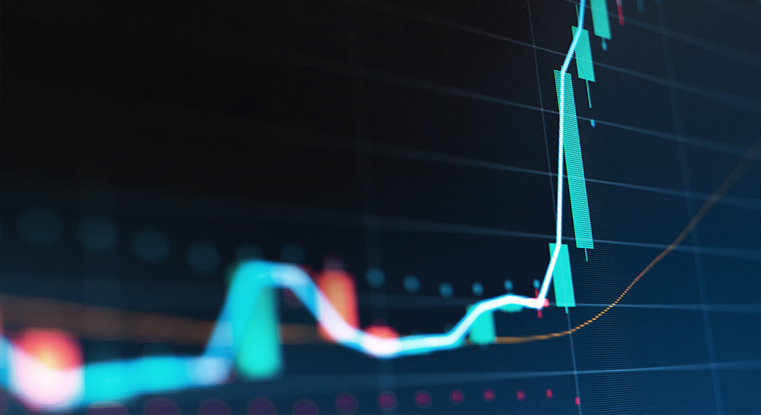
When the music industry revolved around physical media, label owners seeking new artists, promoting new acts, and predicting new hits based their decisions on limited data: including single and album sales, ticket sales for concerts, and word-of-mouth. But since the boom of streaming, the industry has an abundance of data at its disposal, and it all feeds the powerful algorithms behind our streaming services.
These algorithms go far deeper than just the number of streams an artist or song receives. Spotify itself revealed it tracks hundreds of different metrics from users’ listening experiences, including: “what you’re listening to and when, which songs you’re adding to your playlists, the listening habits of people with similar tastes,” and much more. The platform also considers less obvious metrics, like the time of day, the order in which users listen to songs or podcasts, and the release dates of specific songs.
Together, these metrics replace the role of the traditional decision makers in the industry; dictating which songs chart, which artists succeed, and the genres certain demographic listen to. And when you take a deeper dive into the models behind these algorithms, it becomes clearer how they produce such accurate results.
The perfect playlist – curated with big data analytics
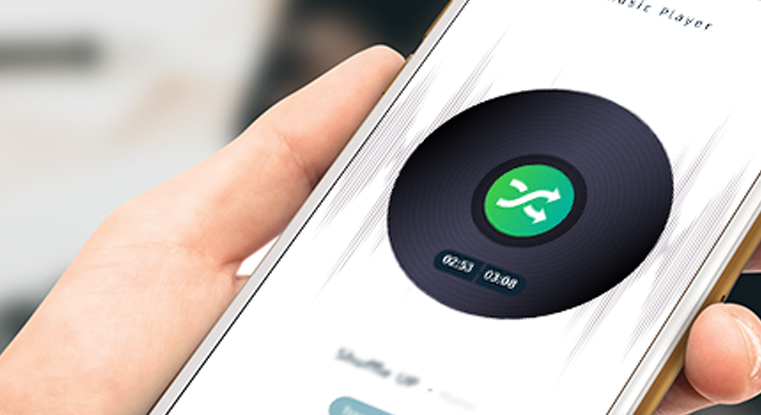
Like Netflix in the TV streaming industry, Spotify’s algorithms are considered as the gold standard in the music streaming industry; and its ‘Discover Weekly’ playlists offer the perfect template to explore these algorithms in action.
Discover Weekly playlists are unique to each user, offering a personalised, curated selection of new songs every week, based on three key machine learning models: collaborative filtering, natural language processing, and raw audio-based models.
Collaborative filtering compares user behaviour to that of other users, similar to traditional predictive algorithms used by platforms like Amazon and YouTube. But in Spotify’s case, with the absence of a star-rating system, the music streaming platform analyses indirect feedback, including the number of times a user has played a specific song and how often they click on an artist’s page.
Combined with natural language processing, Spotify’s algorithms reach an even greater level of accuracy. Spotify’s AI analyses text across thousands of different sources – like articles, tweets, and online forums – capturing the opinions and news surrounding music artists on its platform. Using this information, the platform’s algorithms can tag songs with specific metadata that categorises the demographics they’ll appeal to and the playlists they’ll be suitable for.
Finally, Spotify’s raw audio models help ensure that new songs by artists that haven’t gained any online traction are still discovered. As Sophia Ciocca, Software Engineer at Spotify explains, the platform’s raw audio models analyse songs using convolutional neural networks. These networks identify specific characteristics in raw audio files, including time signature, key, mode, tempo, and loudness.
“Ultimately, the reading of the song’s key characteristics allows Spotify to understand fundamental similarities between songs, and therefore which users might enjoy them, based on their own listening history,” says Sophia.
The unpredictability of predictive algorithms
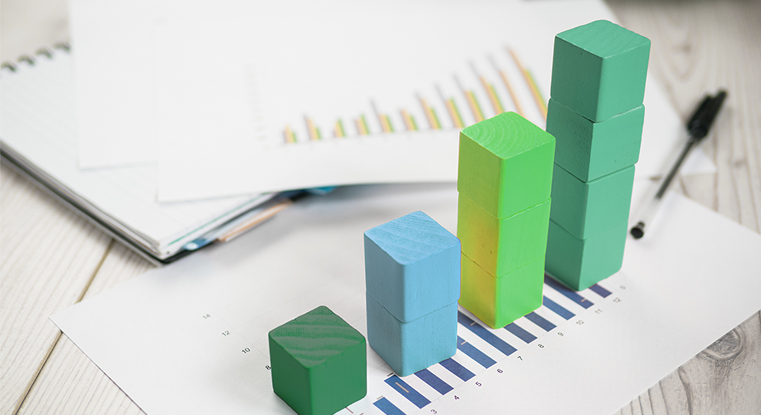
For a platform that’s built on predictive algorithms, you’d think it would be easy to craft the perfect hit based on the characteristics Spotify’s models prioritise – but it’s far from the case.
Spotify’s algorithms generate thousands of unpredictable patterns across its platform every week, creating unexpected listening behaviour within its user base. One famous example is from the 90’s alternative indie band Pavement, whose 1997 B-side track ‘Harness Your Hopes’ saw a sudden spike in popularity over the span of a few weeks in 2017. The song was never released as a single, and didn’t generate much popularity upon its release – but thanks to Spotify’s algorithm, it now ranks on the artist’s page above their chart-topping 1994 single ‘Cut Your Hair’.
This unpredictability hasn’t stopped artists trying to beat the system, however. There are still those that attempt to produce tracks that appeal directly to the algorithm. As coding experts Northcoders explain, “it’s common for tracks to feature the hook, guest artist or a prominent sample in the first few seconds, contributing to a phenomenon known as the ‘Spotify sound’, something that artists are paying a lot to try to procure.”
The concept of the ‘Spotify sound’ has even led to initiatives like the Pandora Music Genome Project, which analyses more than 450 musical attributes in songs and matches them to others in their genres and sub-genres – ultimately, to identify what goes into a hit record.
Technology like this might offer artists some general guidance on what their music should sound like if they want a chance of charting, but we’re not quite at the stage where algorithms can identify the exact ingredients for success.
However, it may only be a matter of time.
Analytics at The Smart Cube
Here at The Smart Cube, we offer bespoke, end-to-end analytics capabilities, from data engineering through to reporting and visualisation, and advanced analytics.
To read about some of the ways we’re helping our clients, or to learn how we can help you achieve your own business goals, visit our analytics and digital solutions.