Our team has been hard at work selecting AI models capable of improving commodity price forecast accuracy. Here’s what we’ve learned.
In a recent blog post, Sidharth Kalia explored Artificial Intelligence’s potential to transform commodity insights for producers, traders and consumers alike, and what companies can do to determine the right commodity intelligence solution for them.
He discussed a variety of organisational demands and factors that can help drive that evaluation process. But how did we reach that point? What did The Smart Cube do to identify those relevant use cases and prove the potential value of narrow AI across commodity price forecasting?
To find out, we spoke to The Smart Cube’s Senior Specialist Anuj Madaan. Here’s what he had to share.
Hi Anuj. Of all the intelligence processes and workflows that could potentially be transformed by AI, why have you chosen commodity price forecasting as a key focus area?
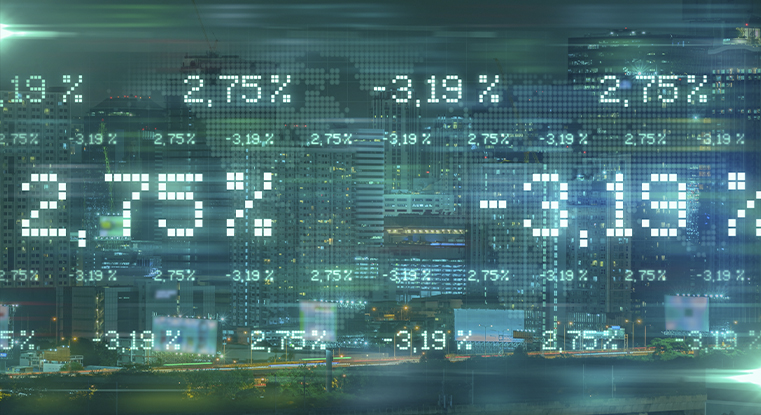
Anuj: Commodity price forecasting is a crucial process for a huge range of organisations. The decisions made using price forecasts directly impact a company’s bottom line, and influence everything from supplier relationships to business continuity. So, it’s an area that demands a very high accuracy of insights and intelligence.
For key commodities like crude oil or gold, there’s also a huge number of levers and metrics to consider that can influence prices. On paper, that makes them an ideal use case for narrow AI – an analysis workload that demands a lot of manual effort, and involves the processing of immense data volumes. For other commodities where data volumes are lower and the relationship between the commodity and its drivers are not always linear, AI techniques help in identifying hidden patterns or relationships to generate accurate forecasts.
The other big reason is that it’s an area one of our biggest pharmaceutical and consumer goods clients wants to improve. By working with them, we were able to look at these models and capabilities through the lens of their organisation, and elevate our work from theoretical exercises, to live, practical changes to help manage commodity price volatility in a real business environment.
What has the team’s work in this area looked like so far? And what have you been able to achieve?
Anuj: To produce accurate forecasts, you need two things: reliable, well-curated data, and a robust AI model to interpret it and turn it into actionable outputs. Thanks to the great work of our commodity experts, we already have plenty of strong data, and a consistent flow of reliable market intelligence coming into the organisation, so we focused our efforts initially on testing lots of models to find the most suitable ones.
The team ran tests using numerous Random Forest, Neural Network, and SVM models, along with sentiment analysis, analysing the outputs of each one to determine which were capable of producing the most accurate forecasts using historical data.
After successfully determining the models best suited to the needs of our clients, we began feeding them with consolidated market intelligence, seeing how they perform in a real commodity price forecasting scenario.
What exactly does the team look for throughout that process? What does a successful model look like?
Anuj: The short answer is that we’re looking for accurate projections of price movements and trends. But in practice it’s a bit more nuanced than that, because there are different types of accuracy to consider, each relevant for different stakeholder groups.
We need to ensure the highest possible levels of absolute accuracy, so procurement teams can utilise forecasts with confidence, and apply them to budgeting and to negotiate the best prices for the commodities they need. But, we also need to see high levels of directional accuracy – how well our models predict the trending direction of prices – for hedging purposes.
Ideally, we’re trying to find models that are capable of delivering both simultaneously, to meet the diverse needs of end users across our clients’ businesses.
How have the models the team selected performed so far? Do they offer a clear accuracy advantage over traditional regression modelling?
Anuj: So far, our augmented AI models have delivered the best results when generating forecasts across shorter time frames. In a test that modelled projected price trends for brent crude oil across a 3-month period, the AI models delivered up to 92% absolute accuracy, and 83% directional accuracy – proving their insights reliable enough to act on with confidence.
But, it’s worth noting that even in areas where AI models haven’t delivered any significant accuracy advantage over manual regression modelling, there’s still a widely-relevant advantage to using AI – reduced manual effort.
If two methodologies produce similar results, but one takes far less time to execute and can deliver those results faster, that in itself is a powerful source of value for many organisations. This is also a relevant consideration if a client is mainly focused on forecast accuracy and is not interested in knowing the individual impact of each driver on commodity prices.
Narrow AI is a growing area of expertise for The Smart Cube. But where does the category and commodity expertise we’re best known for factor into this kind of solution?
Anuj: What we’re doing is AI innovation in the truest sense of the term, but we simply couldn’t achieve any of it without the commodity expertise and deep experience held across The Smart Cube team.
It’s the experience and knowledge of our people – such as knowing the right datasets for each commodity and having a thorough understanding of the commodity value chain – that makes these kinds of advanced AI projects possible. That’s what enables us to select the best models, make decisions based on the context that each model will theoretically be deployed in, and consolidate and optimise the market intelligence that’s combined with each model to generate final price forecast projections.
It’s a great example of how Artificial Intelligence (AI) plus Human Intelligence (HI) results in intelligence accelerated.
So, this isn’t the beginning of a shift towards narrow AI at The Smart Cube?
Anuj: Far from it. Our approach has always been about finding the best use cases for Artificial Intelligence and Human Intelligence in the unique contexts of our clients, then applying the right balance of the two to best meet their needs.
It’s that approach that makes projects like this really successful. We’re not trying to discredit traditional modelling methods and position AI as the outright ‘best’ solution for forecasting. We’re simply trying to find specific use cases where it offers clear advantages, so we can better understand where and when to apply it for our clients.
If our tests found that AI didn’t deliver greater forecast accuracy in any scenario, the exercise wouldn’t be considered a failure, because we don’t have an agenda one way or the other. All we care about is improving forecast accuracy, and finding the best ways to provide our clients with the most reliable insights at the right time.
Learn more about The Smart Cube’s AI+HI approach – and see it in action
AI-based commodity price forecasting is just one of the many innovations helping us find the ideal balance of AI and HI to meet our clients’ exact commodity and procurement insight demands.
You can learn more about our unique AI+HI approach, and see what it could do for your organisation in our new report, How The Smart Cube is empowering Procurement to become the #1 value creator in the business.
If you’re interested in advanced commodity forecasting, read more about our Commodity Intelligence solution.