Artificial intelligence can transform commodity insights for producers, traders and buyers alike—but how can you be sure it’s the right choice for you?
Over the last few years we’ve seen artificial intelligence being used for a host of procurement applications, from spend analysis to supplier risk management. Now, the same technology is increasingly being applied to commodity forecasting.
In the right situation, this can provide invaluable insights. AI makes it possible to look at larger, more complicated data sets over a longer period of time, helping to improve the accuracy of predictions and supercharge decision making, near real-time.
However, while commodity producers and traders are both investing heavily in this technology, commodity buyers are lagging behind.
In this blog, we look at what AI commodity forecasting involves, who’s using it and who isn’t, and how you can tell whether or not it’s the right investment for you.
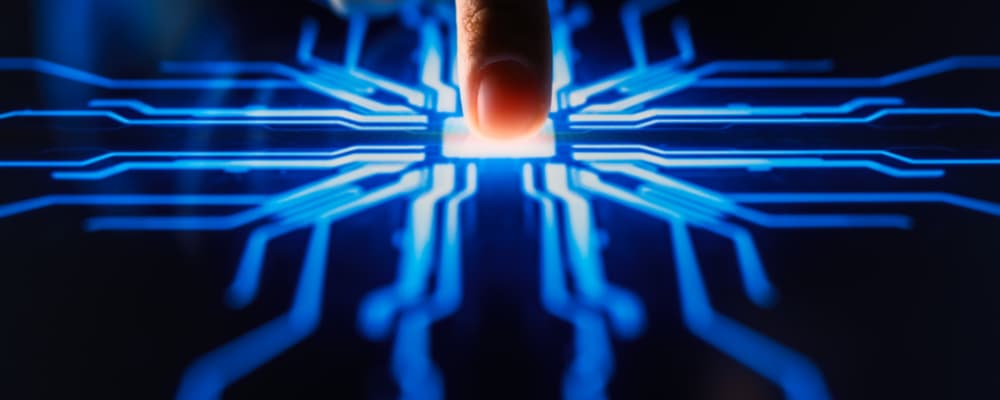
The two technologies behind AI forecasting
AI forecasting in commodities normally involves the use of natural language processing (NLP) and machine learning (ML) to automatically break down structured and unstructured data and create models that predict commodity prices with minimal human intervention.
In doing so, things that otherwise wouldn’t be apparent to the human eye can be brought to the fore, making it easy for producers to predict production, traders to forecast pricing, and buyers to plan more strategic sourcing.
NLP uses rendered algorithms to interpret written data, fuelling techniques like sentiment analysis to garner insights from news articles, emails, and social media posts. It’s often used by traders to analyse current events and anticipate changes in markets.
ML, on the other hand, involves algorithms that can be trained over time to act and think like humans with the goal of improving forecasting. A ‘supervised learning’ approach means that as these algorithms are exposed to more sources, specialists training the models can ensure their continual improvement.
At The Smart Cube, we use a combination of supervised learning algorithms and techniques, namely random forests, support-vector machines and boosting algorithms to forecast commodity prices.
Producers, traders, and buyers: Who’s leading the AI race?
There are three main audiences when it comes to AI solutions in commodities: producers, traders, and buyers. For the purposes of this blog, we are focusing on the procurement function, so we’re leaving investment funds out of the picture for now.
Today, commodity producers are increasingly adopting AI to improve decision making and forecast output. For instance, large farming corporations are incorporating things like Geospatial AI, soil analysis data, and weather data (satellites), to better predict crop yields. Similarly, oil companies are using satellite data to gain a real-time view of tanker locations and storage capacity, and how that will impact supply in the immediate future.
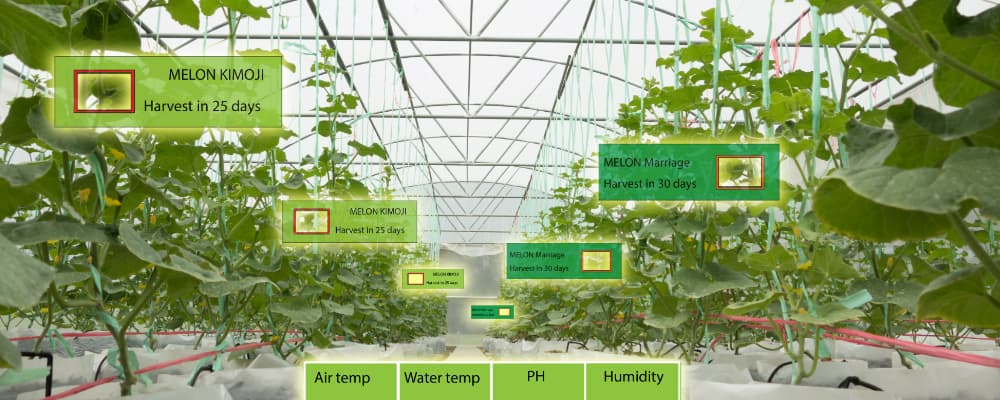
Elsewhere, traders are using their existing experience with high-frequency algorithmic trading in their commodity risk assessments, adopting NLP and ML to make faster and more accurate decisions based on market shifts, traded volumes, market sentiment (chatter) and intraday price movements.
In April 2020, we saw these speculative algo-trading systems cause the WTI crude market to trade at negative $37/bbl. We’ve seen similar commodity price upsets, more recently with the negative FX sentiment (from covid-19) pushing sell-offs in agri-commodities when supply data indicates otherwise.
For companies with the right financial resources, these AI investments have provided vital insights. But, the end-consumers of commodities—manufacturing companies, for instance—are a bit behind the curve when it comes to AI adoption for commodity forecasting. And this can mean that a certain percentage of their price risk is subject to the whims of the market.
For this reason, your inbox is probably overflowing with AI companies offering their services in this area. But rushing to the latest tech might not always be the best approach.
Three questions to ask before you invest in AI
AI can be a powerful tool for commodity forecasting and commodity risk management. It can be the key to making better, faster purchasing decisions, budgeting more accurately, predicting risks and opportunities, and controlling costs. But there’s still a place for traditional methods, too. In fact, in some circumstances traditional modelling may provide a greater advantage than a knee-jerk investment in AI.
The question then, is how do you know whether AI provides the right capabilities for your forecasting needs, or if you should continue with the proven processes you know?
Before you make any decisions, you should ask yourself a few important questions. Firstly, what is your remit for commodity risk management and how much control do you have to manage your commodity-linked exposure?
If you’re only reviewing commodity information every six months to a year and are locked into long contracts, then an AI investment won’t provide much benefit.
However, if you have shorter buying cycles, or contracts that can be updated in line with commodity information, then it could provide the perfect ammunition for better negotiations. In fact, you may even choose to change your buying cycles if you decide that AI could provide enough timely information to make that worthwhile.
The other thing to think about is whether you’re considering the use of AI for budgeting or hedging. If you’re only interested in gaining insights into set price direction, then multi-linear regression modelling (and other traditional forecasting methods) will still prove incredibly effective.
Finally, it’s important to look at what data is available to support any AI investments you might make. The more data you can feed an AI algorithm, the more effective it will be. So, is there enough data available about your commodities to make investments in AI worthwhile? And do you have access to the right external sources? Or would you be investing in an engine you don’t have the fuel to power?
For example, an AI forecasting model of Brent crude oil will map historical crude futures with +200 cost drivers (macro-economic data, FX, bond yields, equities, exchange traded volume, other asset classes, derivative demand, supply & demand, etc.) and will be complemented with daily sentiment analysis (geopolitics, natural disasters, social media, etc.) to predict a price move. But not all commodities have globally-recognised benchmarks and traded contracts, or even fundamental data/news, which means AI can’t be applied in all cases.
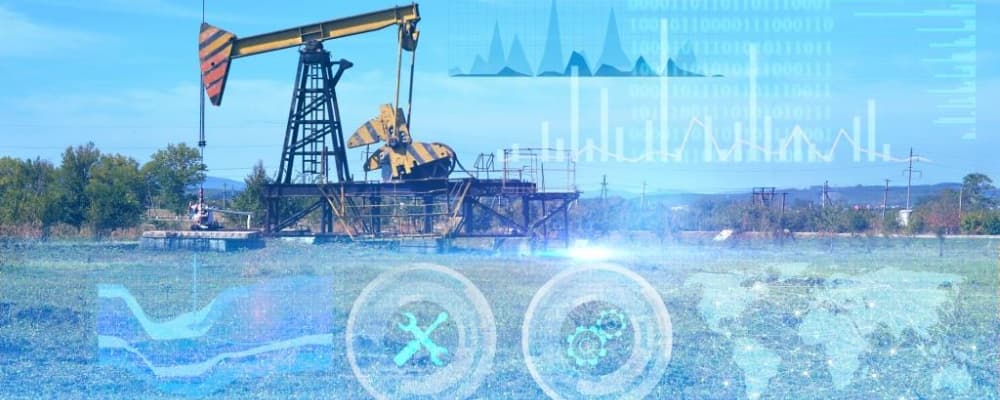
The future of AI in commodity management
With greater volatility and uncertainty affecting commodities, and more data sources available to support decision making, one thing is clear: AI will play a huge role in the future of commodity intelligence. And that’s why there are currently no end of organisations offering AI solutions for commodity managers. We should know, we’re actually one of them.
However, we also know that chasing after the latest tech isn’t always the right thing – adoption, applicability and ROI come into play – and hence traditional approaches to commodity management still provide a huge amount of value.
At The Smart Cube, we offer both traditional and AI-driven commodity intelligence solutions. But first and foremost, we recognise the importance of identifying which one is the best fit for you.
To do this, we embed our experts as a part of your team to gain an understanding of your needs, and suggest the best approach going forward. That might mean the immediate incorporation of the latest AI technologies supported by our experts, or the continued use of traditional methods and a development roadmap for the future. No two cases are the same, because no two companies are.
Read more by The Smart Cube to learn more about how we can help take your commodity management to the next level.